Growing Data Teams from Reactive to Influential
Measuring progress towards company-wide data maturity
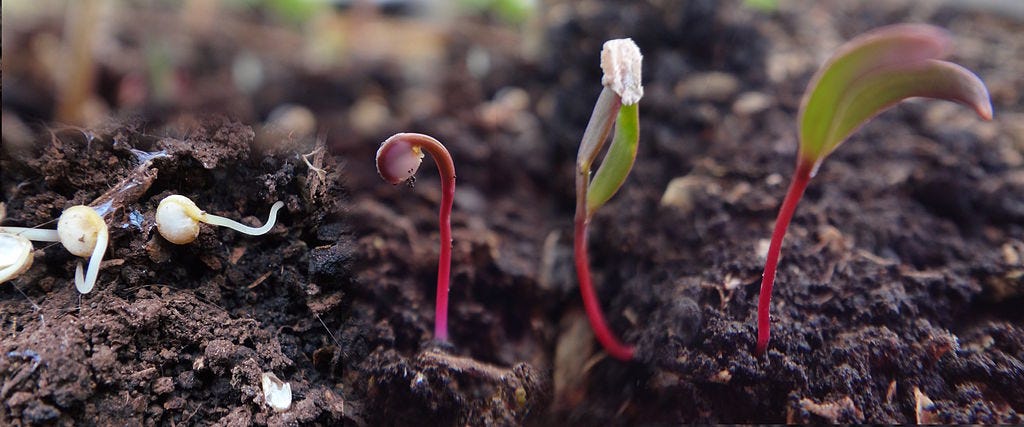
I’ve been on the job hunt recently1, and while interviewing can be an emotionally draining exercise, it’s been really fun to make new connections with data teams of all shapes and sizes and to learn about their current challenges.
When it’s my turn for questions in an interview, I often ask potential direct reports what kind of support they need from their manager to help set them up for success. A common theme I’m hearing (and it’s not a new one2) is that they feel like they are under water; lots of requests are coming in to the data team, they’re not sure what to prioritize week-to-week, and they feel like they’re spinning wheels more than making an impact on the business.
With the exception of a few companies that were founded with data as core to their culture, most places are somewhere on the road to becoming more “data-mature”. This manifests in a structure where the company’s data expertise is heavily centralized on the data team, while decisions about business priorities are not. As the experts on how to best leverage data, it falls on the data team to change the culture, but starting from a position with very little agency to make that change. The data practitioners continue to try to provide their stakeholders with what they ask for in the hopes that company-wide data literacy will someday come.
So the requests pile up, and the team works reactively on data questions that may or may not be best-framed for real business impact. The impulse they feel to push back with prioritization processes is a symptom of being an intrinsic part of the very culture they are trying to change, and all of the negative traits that come with it.
As a manager, I’ve used a framework that defines reactive, proactive and influential stages to describe a data team’s positioning during a broader company-wide march towards data maturity. Using stages to describe data maturity isn’t new,3 but the frameworks I’ve seen are mostly applied at the company-level rather than the data team, and many of them miss giving tactical advice for how to move from one stage to the next. In my experience, it takes more than a new prioritization process to get out of that reactive downward spiral.
Culture change doesn’t happen overnight, and while you’re in the middle of it, it’s hard to feel like you’re making progress. Similar to setting measurable goals in product development, setting explicit goals for data maturity forces us to quantify how much progress we’re making towards it. I’m going to talk about how to help the team make progress, and timescales on which I’ve seen that evolution happen.
Reactive stage: It starts by measuring a baseline
The first thing to do is take the data team’s feeling of being underwater and turn it into something quantitative. Figuring out where requests are coming from and centralizing them into a project tracking system adds some extra process upfront, but it’s invaluable to get that visibility. Some examples include encouraging the team to route requests into a central chat channel rather than private DMs, and to use a ticketing system to manage a transparent backlog, making sure it stays updated when requests are finished. A backlog tool can be useful to show leaders of stakeholder groups what kinds of requests are coming in from their teams, as they might not necessarily have that level of visibility themselves.
Seeing the landscape of requests is a good start, but in order to drive real change in the way things operate, it’s important to know how those analysis results, experiments, dashboards, and other data artifacts are being used to make decisions. It should never be the data team’s job (or the data team manager’s job) to prioritize all of the incoming requests by themselves. In an ROI equation, the data team only knows the “I” with certainty, and can only speculate what the “R” might be if they don’t know what the higher level goals of their business partners are.
When I started at Mozilla in early 2019, the data team was manually designing and analyzing bespoke A/B tests. I ran a survey on roughly 40 experiments completed in the first half of the year and asked the data scientist assigned to each of them what the outcome was. Not all experiments needed to have a significant result to be deemed useful, so examples of “good experiment outcomes” included “a decision was made to ship or not ship a change”, “we learned what was intended”, or even “we found technical issues with the experiment deployment software”, which would enable us to build a better system. The bar for an experiment having been worth doing was fairly low.
When I looked at the survey results, almost half of the experiments had net negative outcomes, including some experiments that were shipped after the business decision had already been made (the “I’ve checked the data box” fallacy). The majority of negative outcomes, however, were that the data scientists weren’t sure what the results were used for after the analyses were completed, indicating that they were accepting the work without question when an experiment request came in. Seeing that a bulk of our work was done with no connection to the impact it had helped us understand that we weren’t having the right conversations with our business partners. After a few months of coaching the team, the business motivation behind the ask was known for almost every analysis.
Proactive stage: Focus on near-term wins for cultural change
Once you have a clear view of where requests are coming from and what they are attempting to solve for the business, you might instinctively start prioritizing based solely on ROI for business impact. But this doesn’t immediately gain you a seat at the table with the decision-makers, and in fact, might have the opposite effect. Stakeholders who feel that they are constantly being told “no”, even when their data partner says it’s in the best interest of the company, will start to feel like the data team isn’t on their side. Making cultural change a goal itself means that there are intermediate steps between being a reactive data team and being a data team with influence.
As you prioritize tasks, rather than only thinking of incoming requests as either impactful to the bottom line or not, think about adding in an unwritten third “culture-changing” category, where the goal is to improve data literacy through learning opportunities for the stakeholders. The work may be the same, but the intention and definition of success changes. Now there are three knobs to turn when determining how much capacity you want to allocate to requests:
Impact-driving (prioritize these)
Culture-changing (still prioritize some of these, especially while in the “proactive” stage)
Non-impact driving or culture-changing (de-prioritize these)
Over a few quarters, the number of impact-driving requests should increase, because the stakeholders have been given the opportunity to gain better intuition for the kinds of analyses that drive real business outcomes.
Getting to the influence stage and longer-term success
Once you have a more healthy flywheel going with the inputs and outputs of your backlog, the data team will inevitably start finding more pain points in their workflows. The next obvious step is to build longer-term foundational work into the team’s capacity. It can be hard to push back and make that time, but managers have many tools at their disposal. For example, when writing a new charter for a particularly reactive team, I explicitly added a line about foundational work to be done, and shared this with the broader organization.
Data teams tend to be a fairly scrappy bunch, and often default to rolling up their sleeves and building what they need in order to get unblocked. But there is an opportunity here to start influencing roadmaps on other teams. Rather than filling in the technology gaps themselves with messy workarounds, my team’s charter also prescribed that they make technical recommendations to the teams we depended on.
Because the data team was now required to proactively drive the conversation, they made the time to work with partners and propose cross-functional solutions. Foundational work was considered part of the backlog of “impact-driving” work, which led to specific quarterly goals, and progress was tracked just as every other initiative owned by the data team.
The approach for prioritizing impactful work in our own backlog depended on how much control we had over solving it ourselves. We broke the work down into three categories:
To do: We have all the data and infrastructure we need to solve this business problem efficiently, but we just haven’t done it yet.
→ Solution: Put it on our data team’s roadmap.
To build: The technology that enables us to solve this business problem in a straight-forward way hasn’t been built yet. For example, we might need new telemetry instrumentation to collect data that we don’t currently have, but it’s something that an engineering team could help us build if they prioritize it.
→ Solution: Work with business partners to put it on their roadmaps.
To discuss: We could build (or buy) the infrastructure, but it’s likely going to need some approvals from higher up. For example, maybe the foundational work is a high priority for us, but too costly of a task to simply get on another team’s roadmap. Or maybe there is data that we haven’t collected before due to some historical policy constraints, and it’s worth revisiting.
→ Solution: Create a written proposal outlining the problem with ideas for solutions, and use it to drive a conversation with the decision-makers.
There will always be a limit to how much can get prioritized, but having this framing allows the team to shine a light on conversations that need to happen. Talk is cheap, and rather than waiting for years (or never) to get foundational work done, progress can be made in a matter of weeks or months.
Finally, acknowledge the positive cultural change
While all of this is happening, you might start to notice some positive feedback trickling in. As data literacy expands outwards from the data team, the impact of this culture shift will start to percolate up to leadership. I started hearing more and more comments like these from senior leaders in the company:
“I love this meeting where we discuss experiment results, I’ve learned so much”
“I can’t live without this product launch dashboard, I used it all the time in my meetings with the SVP”
“That analysis was invaluable to our product’s roadmap”
“My team is starting to self-serve their own data questions now”4
It’s important to bring any signs of positive cultural transformation back to the data team; they did the hard work, and should celebrate that success just as they would for a data analysis that influenced decisions. I kept a running log of quotes and repeated them in bulk during a quarterly data team week talk, less than a year after that same data team was in full reactive-mode. They filled the slide.
For further reading, here are some articles I’ve found useful about increasing the data maturity of a company by evolving data teams from reactive to proactive. Happy to add to this list if you have more links to share:
Mode: Data Maturity Model Guide even has a survey you can take to assess how data-mature your organization is.
Data Kind UK: Moving from Reactive to Proactive Data Work by Bridget Suthersan has some good tips for how to break out of the reactive loop.
Atlan: It’s Time for the Modern Data Culture Stack by Prukalpa Sankar defines a “data culture stack” and steps you can take to drive culture change.
Opinions expressed here are my own and do not express the views or opinions of my past employers.
Shameless plug. Will Lead Data Teams For Food.
This article by DJ Patil about building data science teams was written over 10 years ago, but some of the challenges remain the same today:
“Interaction between the data science teams and the rest of corporate culture is another key factor. It’s easy for a data team (any team, really) to be bombarded by questions and requests. But not all requests are equally important. How do you make sure there’s time to think about the big questions and the big problems? How do you balance incoming requests (most of which are tagged “as soon as possible”) with long-term goals and projects? It’s important to have a culture of prioritization: everyone in the group needs to be able to ask about the priority of incoming requests. Everything can’t be urgent.”
How many stages does it take to become “data-mature”? Is it three stages? Four stages? Four, but start counting at 0? Five stages? Six stages???)
DJ Patil again:
“The result of building a data team is, paradoxically, that you see data products being built in all parts of the company. When the company sees what can be created with data, when it sees the power of being data enabled, you’ll see data products appearing everywhere. That’s how you know when you’ve won.”
What does culture-changing look like? It’s easy(?) to measure bottom line impact but something squishier like culture is hard