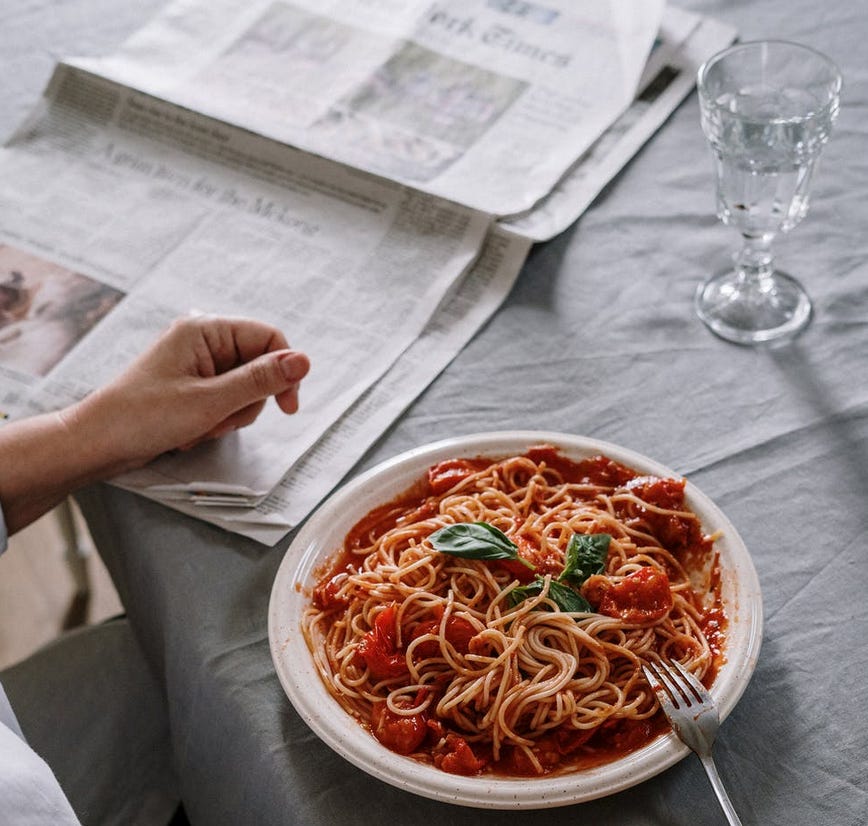
How is your data team situated in your organization?
I’m not talking about the reporting structure, or whether the data team is centralized or embedded within product teams. I mean when someone outside of your org thinks of your team, how do they perceive it?
You may not think about it consciously every day, but your hiring practices, promotion cycles, and ultimately your team’s success are all influenced by how you ideally want the rest of the company to perceive your team. For example, when hiring new data scientists, you might optimize for statistics experts who have experience working with data at scale, have good communication, and can be partners in decision-making. These attributes are all very important on an individual level, but a team is always more than the sum of its parts.
Let’s say you’ve hired an amazing group of data practitioners. How would your stakeholders describe the team, if you were to ask them today? Even with the most highly skilled teams, I’ve heard it all, and it’s not always positive: “a bottleneck”, “too rigorous”, “too slow”, or worst of all, “irrelevant.”
Data teams that sit at the intersection of engineering, product, and leadership work best when they form deep relationships with their business partners. I’ve had the privilege of leading some really amazing data teams, and I’d receive enthusiastic feedback about my direct reports all the time in 1:1s with stakeholders.
While they were raving about the data person they worked most closely with, it surprised me how often stakeholders would simultaneously express feelings that the rest of the data team must be dropping the ball, given that more of their stuff wasn’t getting prioritized. It was almost as though they didn’t understand or could really explain what the data team was responsible for, even though every other data person had a respective stakeholder who felt just as enthusiastic about them.
From a data team manager’s perspective, it can feel like a resource constraint problem; if only each data customer could have two or three more people, they would feel more supported. However, adding more people to the team is a brute-force way to solve the issue1, and in any case, getting extra headcount to double the size of the data team is usually not something a line manager can control.
Zooming out, a data team is ultimately responsible for growing the business through data-informed decision-making. Their true stakeholder is the whole company, even though data practitioners tactically try to achieve success in their day-to-day work by sitting next to individual product owners. Due to the nature of the work that goes into each analysis, a data practitioner and their stakeholder have to spend a lot of time zooming in on lower-level problems, and are not always able to focus on how work across the whole data team connects together to support the business. And this is a problem that a data team manager can do something about.
I had a secret initiative to improve the data team’s brand called “Operation: Spaghetti.” The idea was to try a bunch of different tactics focusing on evangelization, approachability, and trustworthiness. Because it wasn’t clear from the onset which tactics would resonate and which would fall flat, the name referenced the imagery of throwing a bunch of pasta against a wall to see what stuck.2 We tried all sorts of things, including working on a central knowledge repository, driving recurring discussions about analyses with product leadership, and pushing a culture of openness for asking data questions in a “#data-help” Slack channel. All of these tactics were aimed at increasing the transparency of our work as well as the incoming requests to the team, and some had higher ROI than others. I’m sharing some highlights below.
On Evangelization
Back in 2020 I started a newsletter that I sent out to Firefox product leadership about the work our team was doing. The email started out with a quick hello and any relevant announcements (for example, an introduction to a new team hire), followed by highlights from my team’s work. Each highlight had a context-setting headline and at most 2-3 bullet points that described key takeaways from that particular analysis. The highlights were grouped into sections based on different product areas, which made it easy for the reader to find the content they were most interested in.
The reaction to that first email was extremely positive, so I decided to advertise this with the whole product team and invite the rest of the data org to participate. We renamed it “The Data Gazette.”
Initially, I tried to send it out bi-weekly. To collect content for the first few newsletters, I spent a lot of time reaching out to data scientists and data engineers across the org and curating the summaries myself. The client telemetry team already had a great blog named “This Week in Glean” that provided consistent content for The Data Gazette, but I could tell that I wouldn’t be able to maintain the amount of time I was putting into it. I switched to a crowdsourcing model by having the team create their own summaries in a standard “headline with 2-3 bullet points” format, and then asked them to include each summary as a last comment in the corresponding JIRA ticket before closing it. Even if your team doesn’t use a ticketing system, having a consistent format for analysis takeaways makes curating higher-level progress of the team that much easier.
The Data Gazette really turned the tide on how the data team was perceived within the company, and we even received feedback from Product leadership that it appeared the data team was suddenly doing a lot more that quarter, indicating that transparency was indeed a real problem. It was especially loved by leaders within the data organization, because it created a story that made communicating the value of the data org in venues like board meetings that much easier.
On Approachability
I once won a jar of jelly beans in a jelly-bean-counting contest at Fitbit’s San Francisco office. I ended up keeping them on a little table by my desk, and broadcasted jelly bean availability to our team’s stakeholders. Soon enough, Product Managers started to drop by more often, and then stay a while to chat about the challenges they had and how the data team might be able to help them. As I moved into more remote work over the next few years, I missed the days when I could sit face to face with colleagues and connect over candy. It became harder to form relationships over Zoom, but also that much more important.
As evangelization and data democratization unfolds in an organization, data teams are often perceived by non-data practitioners to harbor special elite knowledge that can sometimes unintentionally make them appear unapproachable as humans. I’ve had stakeholders, even at the exec level, who have confided in me that they were afraid to ask certain questions about analyses that might indicate some amount of ineptitude in front of my team; yet, I don’t know of a single data practitioner who believes that there is such a thing as a stupid question.
Stakeholders expect statistical and technical rigor, but it often takes some coaching to get Data Scientists to focus on the things that matter in presentation, especially when building relationships. Sometimes it means compromising on a more advanced or nuanced approach to a data problem in order to let the stakeholder in and have more visibility and control over the analysis. Marissa Gorlick, a former Data Scientist on my team who was exceptionally skilled at creating outstanding relationships with stakeholders, once said “We just need to give them some carrot cake.” She meant that sometimes we have to give stakeholders what they want in order to build that trust, but I especially liked the metaphor of getting them to eat their veggies (statistical rigor) by wrapping it in a delicious confection (who doesn’t want cake?). This is less of a tactical suggestion, but as data leaders, encouraging the team to serve up carrot cake is a healthy cultural shift to improve approachability.
On Trustworthiness
Lastly, building trust takes active work in any partnership. Stakeholders may trust that the analyses built by data teams are statistically rigorous, but do they trust that the data team will deliver results on time? While not every deadline can always be hit, setting the right expectations as early and often as possible will make data team customers always feel in the loop and minimize unnecessary surprises. In the past, I have leveraged tools like JIRA tickets to make sure that weekly updates were available for asynchornous communication. This sometimes led to the added bonus that the number of necessary meetings was reduced, for a double-win.
Building trustworthiness into a data team’s brand also means that if there are concerns with the tools built by the team or even how the team operates, data leaders need to demonstrate visible progress towards addressing them. Just like the iterative feedback cycle in product development, customers will start wanting to rely less and less on a data team if they don’t feel that it is meeting their needs. Having a product-minded approach to data tool development helps, as does leading solutions-oriented discussions with everyone involved.
However you choose to make change, shifting organizational culture is a full-time job. I’d be interested to hear how other data leaders have formed strong relationships with their business partners, so feel free to leave comments below!
Often adding more people to a problem makes the problem worse, as observed by Frederick Brooks in The Mythical Man-Month
My Italian friends would disown me if I encouraged people to literally throw pasta at a wall, so I don’t actually condone this. Also for some reason, a search for “throw spaghetti against a wall” stock images mostly returns spaghetti flung with sauce, which really is going too far.
The "Data Gazette" idea is great, I've been thinking through a similar project for a while. What kind of content seemed to be the most powerful? Did you roll in weekly metrics summaries, or was it more "platform updates", or both?
It is curious how as data managers are getting to the same conclusions and initiatives. IMHO getting trust in data and data products is the most challenging one. I found out that doing small prototypes and assign one data customer/colleague as a PoC gives ownership that eventually translates to trust. Now we are assigning one for each main data project.