Don’t just run your data team like a product team, run it like a company that needs to scale
A minor change in framing.
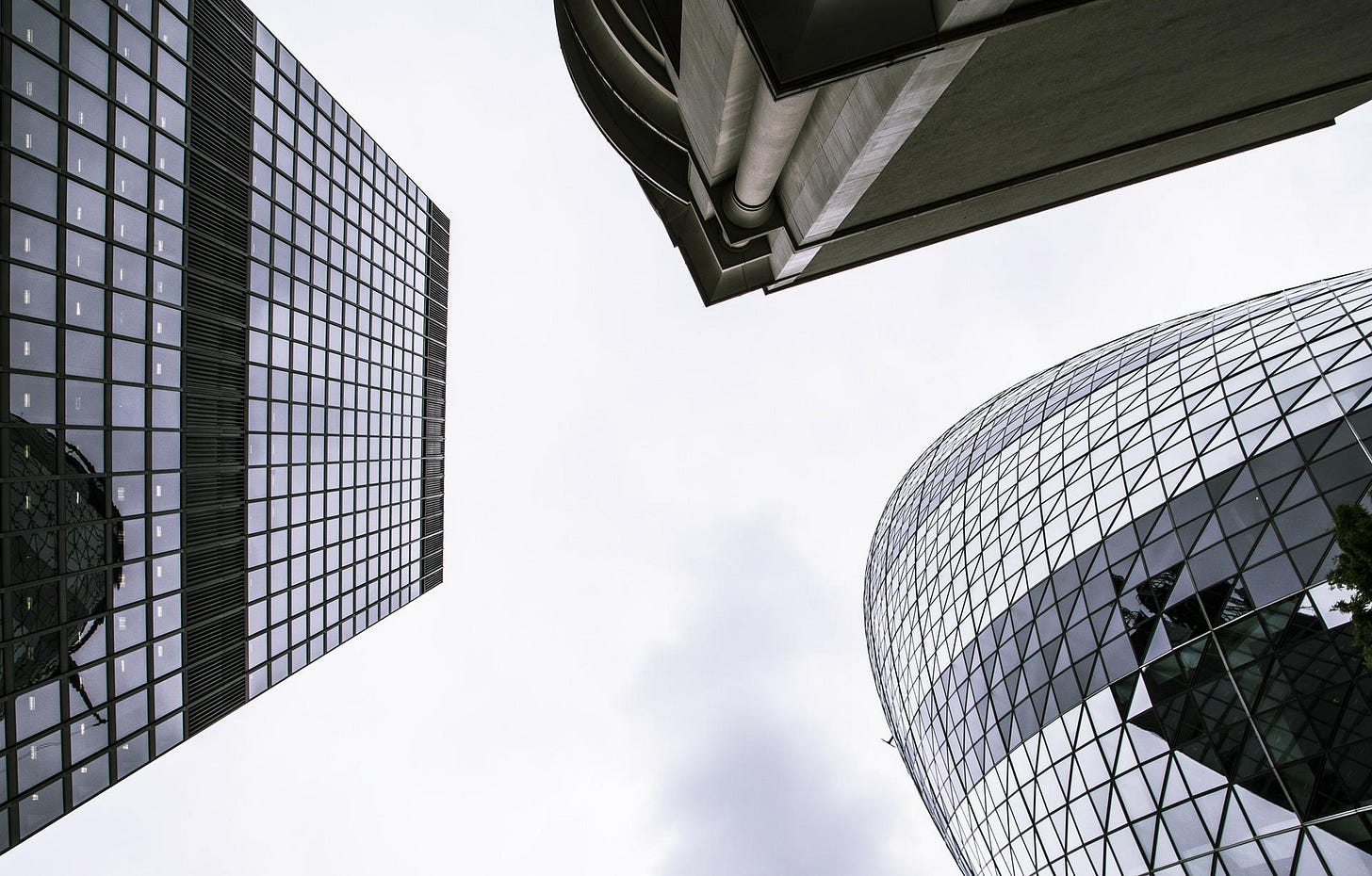
After several rounds of interviews with what feels like countless teams, I’ve finally settled on a new role, and have just about completed my first two weeks at Gusto! Now that my sabbatical between jobs is over, I’ve had time to reflect on what I’ve learned while kicking off this blog. Coming back into the data community discussion circles, having 1-on-1 conversations with data practitioners at all stages of their careers, and hearing feedback about what I have been writing have all helped me evolve my philosophy about what it means to be a leader in the modern dataverse.
One of the first reflections I wrote about was how putting a product lens on analytics tooling helped me prioritize what was important. I should mention that at the time I started writing last November, I had no idea that blogging about data strategy was still a thing, let alone that Benn Stancil was leading “Friday fights” on Twitter, or that Emilie Schario and Taylor Murphy had already written a very thoughtful, very widely-shared blog post about running a data team like a product team, because I was in fact, living under a rock. Welp, at least when it comes to experiencing data (im)maturity, I have since found great comfort in the fact that it’s definitely not just me.
So as click-baity as the title is, I’m not here to challenge the idea that the “data team as a product team” framing is wrong, as much as to evolve the idea with a ‘yes, and…’
I keep coming back to this issue of scale. Data teams are always under-resourced, but simultaneously can be seen by those who hold the purse strings as an already expensive investment. Companies hire data teams to drive growth and results, but even modern data teams are struggling to scale fast enough to support the operational cadence of their organizations. For example, a marketing executive might want to come up with a hypothesis, test it, and get an answer within a very short time frame. If a data scientist always needs to be in the loop, they probably won’t be very successful at keeping up without burning out. Whether or not that short turnaround time actually drives positive business outcomes in every scenario, that’s what stakeholders feel they need — and that they are currently lacking. Business leaders might feel as though they have already approved a substantial investment in data, which creates a disconnect when data teams appear slow to act on the promise of delivering value. Asking those same business leaders to allocate even more resources might feel, to them, like throwing more money on the fire without solving for their imminent pain points. The mid 2010’s were a boom time for data teams, but something needs to change in the way data leaders approach the problem of scale, and they can’t expect funding to just keep coming for free.
Sitting in onboarding, I’ve been learning about the mission of the company, what our advantage is, and how we measure success. I couldn’t help comparing Gusto’s fight for continual rapid growth in the market to the struggles many data teams face while trying to expand data culture at less data-mature organizations. I found myself nodding along each time a struggle was acknowledged and how they are creating focus and alignment to tackle it.
I’m not a founder, but from what I can tell, very early stage companies are all about building the product.1 At some point, other support organizations need to come in and help the founders scale and grow their product into a mature organization. It’s not always the most straightforward path, and I’ve seen founders struggle with that transition, having to let go2 of the thing they loved doing in order to transition from a founder mindset into the role of a CEO. That means bringing in not only new product expertise, but marketing, sales, design, finance, customer support, operations, and community outreach, to name a few.3
My analogy to data leaders is that at some point along our organization’s data maturity journey, we hit a phase where we need to make that same kind of transition, from data product-builders to data CEOs. If we restrict ourselves to the data founder mindset that got us to where we are, we might miss some opportunities to re-prioritize and meet the scaling needs of our internal customers. For one concrete example, I loosely held the strong belief that random, non-business-priority ad hoc requests thrown our way by data-curious stakeholders were a complete waste of time (I even mentioned it in a snide footnote a few months ago). But if I had thought like a sales team rather than a frantic, underwater product-builder, I might have realized that anyone who shows interest in data, no matter what the motivation, is a potential customer. I might try to spend less time falling back on the resource constraint argument, and spend more effort figuring out ways to keep ourselves from having to turn those free inbound customers away. Prioritization is still key, but what is most important may shift and change over time.
In a practical sense, it might mean looking for different kinds of traits and skills as we bring on new data practitoners, ones that may previously have been overlooked. If we need to switch to a scale-mentality, what other hats should we make sure the data team is capable of wearing? Over these past couple of weeks, I jotted down some inspiration from other disciplines that might be worth stealing best practices from:
Marketing - What is our data “brand”? How do we communicate that brand outwards? How do we communicate to our customers when new data capabilities/features are available? How do we convert new data users? How do we uplevel existing data users?
Operations - Are we keeping our data products up and running smoothly? What is considered an “incident” and how do we handle it? How do we do program management, communicate goals internally and align data practitioners with different roadmaps? What could be more efficient? What needs to be built internally vs what can we outsource?
People team - How are we recruiting the best talent on our data teams? How are we keeping our current data team engaged and successful? What does the career ladder need to look like for different kinds of data practitioners? How do we address the pay gap between different kinds of data practitioners? What are our values, and how do we live them every day?
Finance - How do we allocate resources and track them? Do we know our COGS for the data products we are building? What are the best KPIs to represent what “sustainable” looks like?
Product - as I (and others) have mentioned before, product skills are still core to a data team’s success, and thinking about how to measure that success is equally as important.
This is a non-exhaustive list and I haven’t thought through the analogy beyond these first few points, but hopefully it inspires some food for thought and maybe some new subjects to read up on. I’m certainly not a subject matter expert in most of these other disciplines, but I now work again with people who are, and I’m going to use this fresh opportunity to pay more attention to how these and other teams operate, beyond just what I need to know in order to be a good data partner.
Though it does feel like founders are constantly running around trying to secure funding, which is not to dissimilar to what data leaders often need to do.
I’ve kept this article in my back pocket ever since we first discussed it at the startup I worked for several years ago. One of my favorite quotes is “Almost everything about scaling is counterintuitive.”
And data. How many data teams do you know of who actually use data to inform their roadmaps as much as we want our stakeholders to?